An Online Cortical Machine Learning Artificial Intelligence Technique for Drug Discovery
Keywords:
Artificial Intelligence, drug discovery prediction, bioinformatics, DNA sequence, online cortical machine learning, relaxation factor, RP-HPLCAbstract
Bioinformatics deals with the analysis and interpretation of biological data by using tools of information science. Drug discovery prediction which is a process of discovering new candidate medications from some molecular compounds has challenged professionals in the field of medical sciences. Tools that have assisted in drug discovery and have been reported by researchers includes the use of decision trees, induction programming logic, expert systems and supervised neural networks. In this research paper, we propose an approach to the drug discovery and prediction problem using a variant of an unsupervised online cortical machine learning artificial intelligence technique. The approach has an explicit tuning parameter called the relaxation factor used in determining possible new candidate sequence. Experiments on a popular DNA sequence dataset and a reversed-phase high-performance liquid chromatography (RP-HPLC) drug dataset were performed to determine whether the proposed technique can give effective predictions. The results showed that the approach compares favorably with the other methods reported in the literature but has a more promising performance when it is set to lower relaxation values.
Downloads
References
King, R. D., Muggleton, S., Lewis, R. A., & Sternberg, M. J. (1992). Drug design by machine learning: The use of inductive logic programming to model the structure-activity relationships of trimethoprim analogues binding to dihydrofolate reductase. Proceedings of the national academy of sciences, 89(23), 11322-11326.
Hirst, J. D., King, R. D., & Sternberg, M. J. (1994). Quantitative structure-activity relationships by neural networks and inductive logic programming. I. The inhibition of dihydrofolate reductase by pyrimidines. Journal of ComputerAided Molecular Design, 8(4), 405-420.
King, R. D., Hirst, J. D., & Sternberg, M. J. (1995). Comparison of artificial intelligence methods for modeling pharmaceutical QSARS. Applied Artificial Intelligence an International Journal, 9(2), 213-233.
Towell, G. G., Shavlik, J. W., & Noordewier, M. O. (1990, July). Refinement of approximate domain theories by knowledge-based neural networks. In Proceedings of the eighth National conference on Artificial intelligence (Vol. 861866).
Buciński, A., Wnuk, M., Goryński, K., Giza, A., Kochańczyk, J., Nowaczyk, A., & Nasal, A. (2009). Artificial neural networks analysis used to
evaluate the molecular interactions between selected drugs and human α 1-acid glycoprotein. Journal of pharmaceutical and biomedical
analysis, 50(4), 591-596.
Anireh, V., & Osegi, E. (2017). HTMMAT: An online prediction software toolbox based on cortical machine learning algorithm. https://hal.archives-ouvertes.fr/hal-01550944
Hawkins, J., Ahmad, S., & Dubinsky, D. (2010). Hierarchical temporal memory including HTM cortical learning algorithms. Techical report, Numenta. Inc, Palto Alto.Zandkarimi, M., Shafiei, M., Hadizadeh, F., Darbandi, M. A., & Tabrizian, K. (2013). Prediction of pharmacokinetic parameters using a genetic algorithm combined with an artificial neural network for a series of alkaloid drugs. Scientia pharmaceutica, 82(1), 53-70.
Tayarani, A., Baratian, A., Sistani, M. B. N., Saberi, M. R., & Tehranizadeh, Z. (2013). Artificial neural networks analysis used to evaluate the
molecular interactions between selected drugs and human cyclooxygenase2 receptor. Iranian journal of basic medical sciences, 16(11), 1196.
Agatonovic-Kustrin, S., & Beresford, R. (2000). Basic concepts of artificial neural network (ANN) modeling and its application in pharmaceutical research. Journal of pharmaceutical and biomedical analysis, 22(5), 717-727.
Segler, M. H., Kogej, T., Tyrchan, C., & Waller, M. P. (2017). Generating focussed molecule libraries for drug discovery with recurrent neural networks. arXiv preprint arXiv:1701.01329.
Cui, Y., Ahmad, S., & Hawkins, J. (2016). Continuous online sequence learning with an unsupervised neural network model. Neural computation.
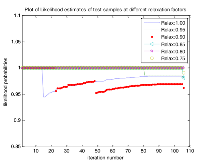
Downloads
Published
Issue
Section
License

This work is licensed under a Creative Commons Attribution-NonCommercial-ShareAlike 4.0 International License.